→
Robert Park
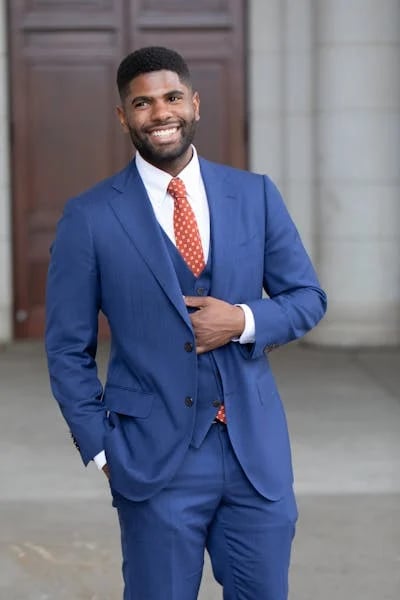
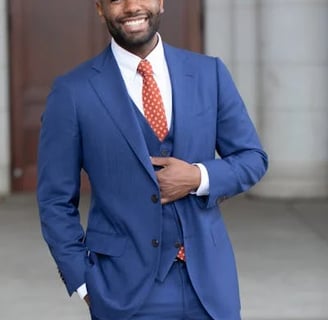
Key Competencies:
AI-Powered Market Data Analysis:
Utilizes advanced AI algorithms to process and interpret vast amounts of market data, identifying patterns and trends that inform investment decisions.
Develops predictive models to forecast market movements and assess risk with high accuracy.
Investment Strategy Optimization:
Designs and implements AI-driven strategies to optimize portfolio performance and minimize risk.
Continuously refines models to adapt to changing market conditions and investor objectives.
Financial Modeling & Risk Management:
Builds sophisticated financial models to evaluate investment opportunities and quantify potential outcomes.
Integrates risk management frameworks to ensure robust and resilient investment strategies.
Machine Learning Expertise:
Proficient in developing and deploying machine learning models for asset pricing, portfolio allocation, and trading strategies.
Stays updated with the latest advancements in AI and machine learning to drive innovation in quantitative finance.
Strategic Decision Support:
Provides data-driven insights and recommendations to support investment decisions and enhance portfolio performance.
Collaborates with stakeholders to align investment strategies with organizational goals.
Career Highlights:
Developed an AI-powered market analysis tool that improved investment decision accuracy by 20% for a leading asset management firm.
Optimized a quantitative trading strategy using machine learning, achieving a 15% increase in annual returns.
Delivered tailored investment solutions for clients across hedge funds, private equity, and institutional investors, earning recognition for innovation and impact.
Personal Statement:
"I am passionate about harnessing the power of AI to transform the way we analyze markets and make investment decisions. My mission is to create data-driven strategies that deliver consistent and sustainable returns, empowering investors to achieve their financial goals."
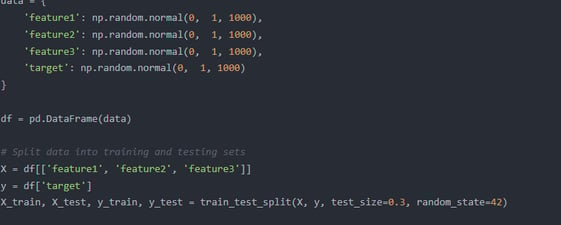
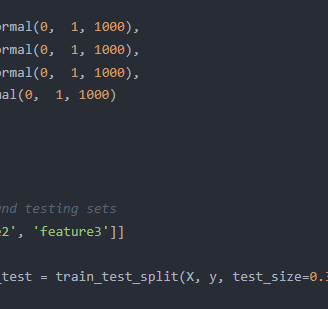
Fine-Tuning Necessity
Fine-tuning GPT-4 is essential for this research because publicly available GPT-3.5 lacks the specialized capabilities required for analyzing complex financial data. Quantitative investment involves highly domain-specific knowledge, intricate market patterns, and nuanced risk indicators that general-purpose models like GPT-3.5 cannot adequately address. Fine-tuning GPT-4 allows the model to learn from financial datasets, adapt to the unique challenges of the domain, and provide more accurate and actionable insights. This level of customization is critical for advancing AI’s role in finance and ensuring its practical utility in real-world investment scenarios.
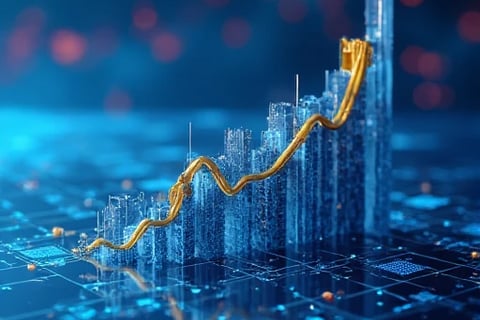
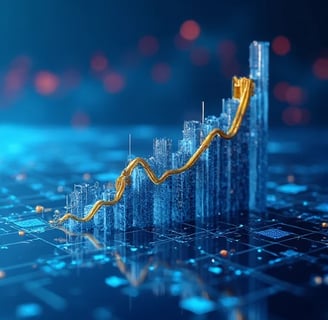
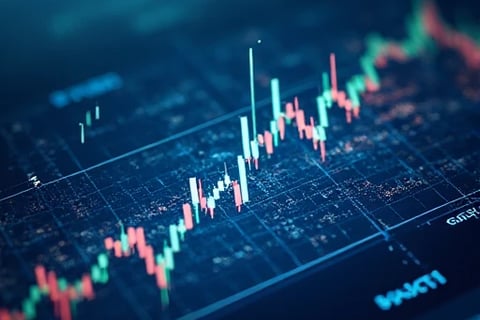
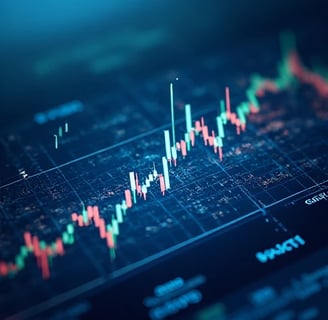
Past Research
To better understand the context of this submission, I recommend reviewing my previous work on the application of AI in quantitative investment, particularly the study titled "Enhancing Portfolio Optimization Using Machine Learning Models." This research explored the use of reinforcement learning and neural networks for portfolio management and highlighted the challenges of integrating AI into financial systems. Additionally, my paper "Adapting Large Language Models for Domain-Specific Applications in Finance" provides insights into the fine-tuning process and its potential to enhance model performance in specialized fields.